Confronting the biases embedded in AI and mitigating the risks
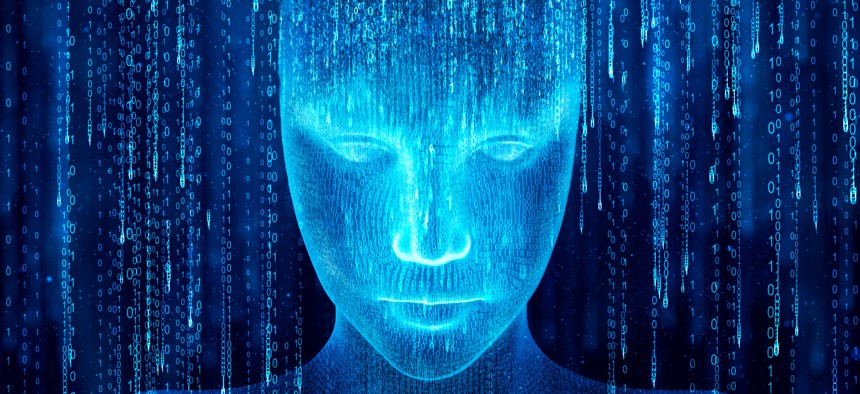
Gettyimages.com/ Yuichiro Chino
The risk of bias is high in artificial intelligence systems and the explosion of AI should prompt a call-to-action to develop best practices.
Race, gender, disabilities, and other biases may be inadvertently embedded in artificial intelligence systems, forcing computational systems to replicate historical problems. The explosion of new AI technology needs a call-to-action from regulators and organizations to mitigate those risks with best practices for AI applications.
Examples of this are testing algorithms for discriminatory outcomes, leveraging the NIST Voluntary Framework for examining AI systems (for issues involving fairness and equity), and studying algorithms and their models for biased outcomes.
Currently, there are no federal laws that dictate the examination and auditing of AI systems. However, these biases were found to be so pervasive, that the Federal Trade Commission in 2021, urged companies to test their algorithms for discriminatory outcomes. This means that organizations should create democratic machine-learning tool sets that embody the ethics and morality of biological social beings, instead of flawed socio-technical models.
For example, it was found that some predictive policing software, disproportionately target African-America and Latino neighborhoods. This proves that a socioeconomic “mindset” was baked into the system, and the model inherited the “brain” and behavior of the humans that compiled them. This is how biases get encoded into technology systems.
There is so much public interest in unbiased outcomes, that it is necessary to rein in these generative AI systems and algorithms with sound policies that eliminate discriminatory and exclusion practices. Industry has proven that computational engines cannot consistently be accurate; however, this emerging technology has such vast potential that public trust must be garnered.
The US government is taking the lead to ensure equitable and accurate AI with the Bias Toolkit - a toolkit that helps government teams understand and mitigate bias in their data and algorithms. This columns discusses the Bias Toolkit and its impact on the elimination of bias in AI.
The Bias Toolkit
Many forward-thinking organizations have been on the algorithmic-accountability front lines serving customers with solutions that investigate, monitor and audit complex AI systems, to ensure equitable and accurate AI outcomes.
The Bias Toolkit is a collection of tools designed to assist in the mitigation of bias in federal data by addressing issues in data planning, curation, analysis, and dissemination. These tools are:
- Algorithm Audits to Prevent Algorithm Harm
The Model Card Generator - A model card is a documentation tool that increases transparency and shares information with a wider audience about machine language (ML), AI, or the automation model’s intent, data, architecture, and performance. It reduces bias in government machine learning workflows by exploring the model’s ability to perform across sensitive classes and by collecting this information in a readable format for a wider audience. This audit-type function provides context and transparency into a model's development and performance for effective public oversight and accountability.
- Natural Language Processing to Ensure Empathy
The Ableist Language Detector - Ableist language is language that is offensive to people with disabilities and can cause them to feel and be excluded from jobs they are qualified to perform. A natural language processing-powered web application was developed that identifies ableist language and recommends alternative language to make the posting more inclusive for persons with disabilities.
- Algorithm Accountability Investigation
The Data Generation Tool - A suite of Jupyter (Python) notebooks that produces synthetic datasets that compare the expected behavior and the actual output of a given ML model. Each notebook addresses a different practical application that may be relevant to “customers’” models. This human intervention can detect a potential bias that occurs when two data observations with the same characteristics are treated differently by the model.
Here are just a few of the Bias Toolkit positive impacts:
- Responsible AI - because biases are no longer reflected in the computational system, organizations can experience more credible, equitable and transparent AI outcomes.
- Trust Cultivation – supports the accelerated adoption of AI within agencies and assists in modernizing operations, thus cultivating trust among users. Also highlights federal agency leadership adoption of trustworthy AI and sets a similar tone in commercial sectors.
- Empathy Indication - creates a framework that facilitates more empathetic thinking thus mitigating potential harm when examining population data sets.
- Risk Mitigation – breaks down boundaries and holds systems accountable with advanced risk management techniques. Also nurtures collective enthusiasm for AI technology.
AI and related technologies are reducing federal data bias in a more effective and responsible way.
Annette Hagood is the director of marketing and strategy for Whirlwind Technologies, LLC. She has extensive industry expertise in government, education, and healthcare verticals for companies like Ricoh, Deloitte and AT&T. Annette has an MS in Computer Science from Howard University. She can be reached at ahagood@wwindtech.com.